- Speaker
- Prof. Paolo Zanardi
- University of Southern California, USA
- Abstract
Extracting useful information from large data sets can be a daunting task. Topological methods for analysing data sets provide a powerful technique for extracting such information. Persistent homology is a sophisticated tool for identifying topological features and for determining how such features persist as the data is viewed at different scales. I will discuss quantum machine learning algorithms for calculating Betti numbers—the numbers of connected components, holes and voids—in persistent homology, and for finding eigenvectors and eigenvalues of the combinatorial Laplacian. The algorithms provide an exponential speed-up over the best currently known classical algorithms for topological data analysis. See also: http://news.mit.edu/2016/quantum-approach-big-data-0125.
Reference: Seth Lloyd, Silvano Garnerone e Paolo Zanardi, Quantum algorithms for topological and geometric analysis of data, Nature Communications 7, 10138 (2016)
- About the Speaker
Paolo Zanardi got his PhD in Theoretical Physics at the University of Roma "Tor Vergata" in 1996. He then joined the newly born field of quantum computation and information working for ten years at the ISI Foundation in Torino. Since 2007 he moved to University of Southern California in Los Angeles where he is now full Professor of Physics. He published more than 130 papers gathering more than 10,000 citations. In 2011 he was elected Fellow of the American Physical Society for his "pioneering contributions", including the introduction of noiseless quantum codes (aka decoherence free sub-spaces) and the invention of Holonomic Quantum Computation.
- Date&Time
- 2016-07-26 2:00 PM
- Location
- Room: A403 Meeting Room
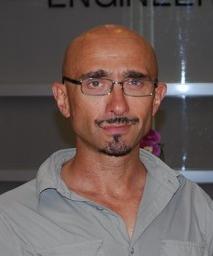