- Speaker
- A/Prof. Tao Zhou
- Academy of Mathematics and Systems Science, CAS
- Abstract
We introduce normalizing field flows (NFF) for learning random fields from scattered measurements. More precisely, we construct a bijective transformation (a normalizing flow characterizing by neural networks) between a reference random field (say, a Gaussian random field with the Karhunen-Lo\`eve (KL) expansion structure) and the target stochastic field, where the KL expansion coefficients and the invertible networks are trained by maximizing the sum of the log-likelihood on scattered measurements. This NFF model can be used to solve data-driven forward, inverse, and mixed forward/inverse stochastic partial differential equations in a unified framework. We demonstrate the capability of the proposed NFF model for learning Non-Gaussian processes, mixed Gaussian processes, and forward \& inverse stochastic partial differential equations.
- About the Speaker
周涛,中国科学院数学与系统科学研究院副研究员。曾于瑞士洛联邦理工大学从事博士后研究。主要研究方向为不确定性量化、随机计算方法以及高维逼近算法等。2016年获中国工业与应用数学学会青年科技奖,2017年获中科院数学与系统科学研究院“陈景润未来之星”称号,2018年获国家自然科学基金委“优秀青年科学基金”资助。 2017年起担任国际不确定性量化期刊(International Journal for UQ)副总编(Associate Editor in Chief)。同时担任Commun. Comput. Phys. 和SIAM J Sci Comput等国际期刊编委,2018年起任国际期刊East Asian J. Appl. Math.执行主编(Managing Editor)。国防科工委科学挑战专题领域一“复杂系统模型不确定性评定方法”首席科学家。
- Date&Time
- 2021-11-11 10:00 AM
- Location
- Room: Tencent Meeting
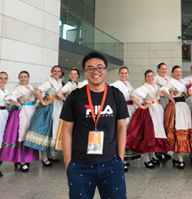