- Speaker
- Prof. Zhi-Jian He
- South China University of Technology
- Abstract
Variational inference (VI) is usually computationally effective compared to simulation-based methods, such as Markov chain Monte Carlo. In many applications typically arising from natural and social sciences, the likelihoods concerning probabilistic models are intractable, but can be unbiasedly estimated. In this talk, I will show how to use VI to approximate Bayesian posterior by a tractable distribution chosen to minimize the Kullback-Leibler (KL) divergence between the posterior distribution and the variational distribution in the likelihood-free setting. To this end, our recent work proposed unbiased estimators based on multilevel Monte Carlo (MLMC) for the gradient of KL divergence so that the minimizer of the KL divergence is obtained by the stochastic gradient decent (SGD) algorithm. On the other hand, we proposed an adaptive mixture population Monte Carlo (MPMC) algorithm to solve the optimization problem within a family of mixture distributions. Differently from the SGD algorithm, the MPMC algorithm relies on importance sampling computations other than the complicated gradient estimation of the KL divergence. This is joint work with Xiaoqun Wang, Zhenghang Xu, Shifeng Huo, and Tianhui Yang.
- About the Speaker
何志坚,华南理工大学数学学院教授、博士生导师,国家高层次青年人才计划入选者。研究兴趣为随机计算方法与不确定性量化,特别是拟蒙特卡罗方法的理论与应用研究。相关研究发表在统计学四大期刊Journal of the Royal Statistical Society: Series B, 计算科学重要期刊SIAM Jurnal on Numerical Analysis, SIAM Journal on Scientific Computing和Mathematics of Computation, 运筹管理权威期刊European Journal of Operational Research等。博士论文获得新世界数学奖银奖。主持两项国家自然科学基金项目以及两项省部级项目。
- Date&Time
- 2022-10-10 2:30 PM
- Location
- Room: Tencent Meeting
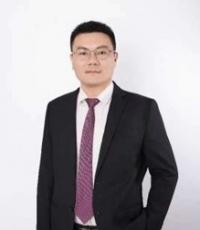